Qualcomm XPRIZE Tricorder 競賽歷史與 HTC 貢獻
Edward Chang and Chung-Kang Peng (reviewed)
2017/03/30 — 台北 · 台灣
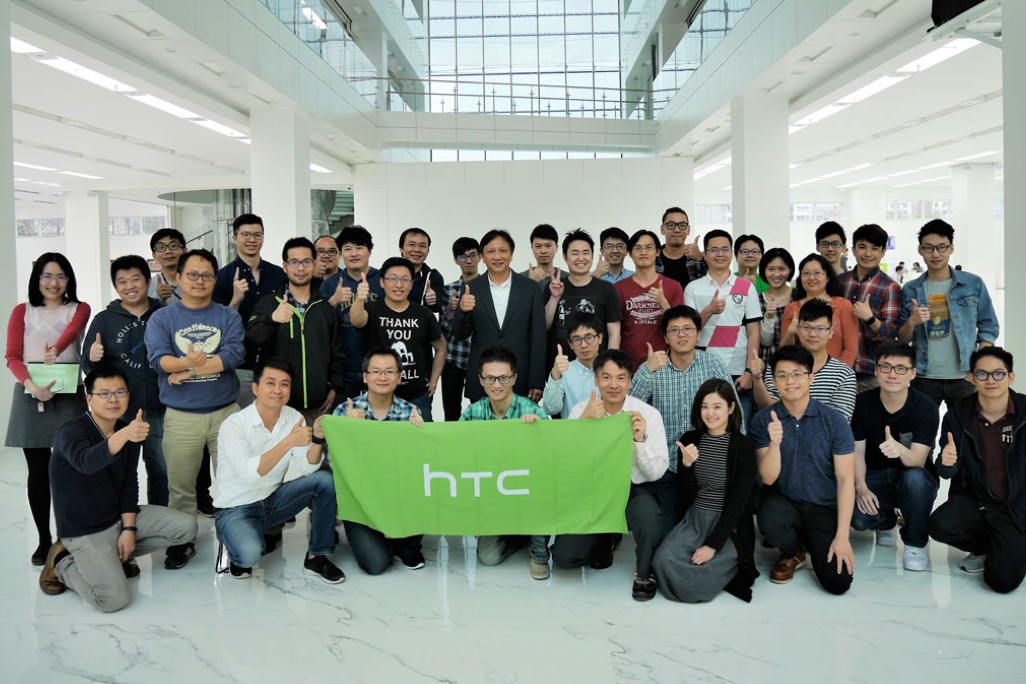
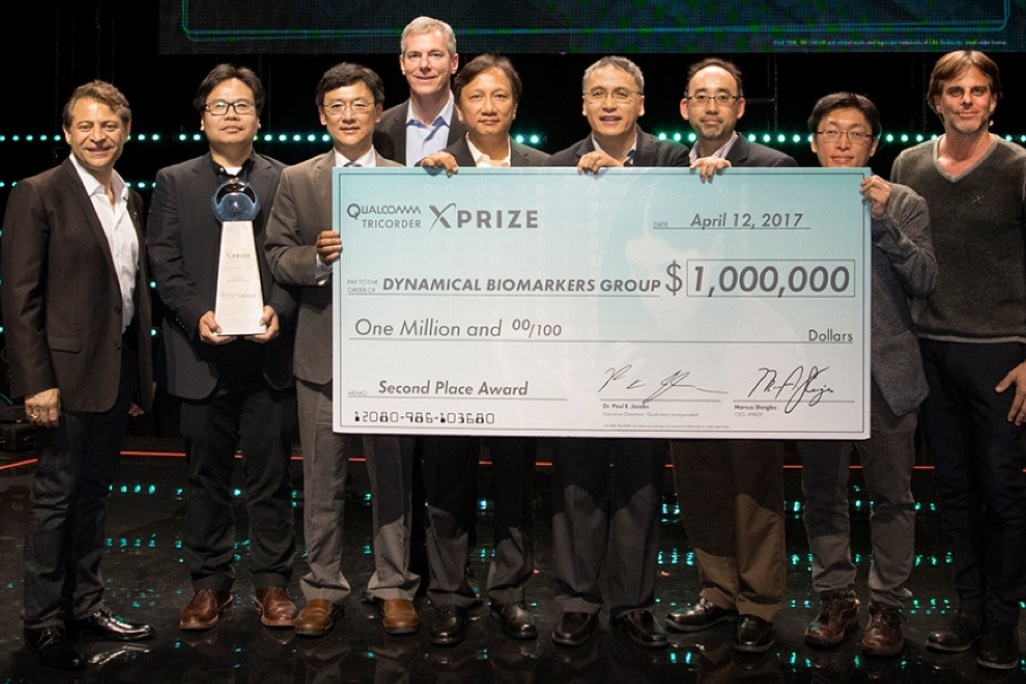
2013 年第一季 XPRIZE 基金會宣布了 Tricorder 競賽,由 Qualcomm 贊助提供獎金共一千萬美元。競賽內容為研發一款五英磅(大約 2.3 公斤)以下的醫療儀器,能夠正確的判別 12 項疾病和連續監測五項生理訊息。此儀器必須能居家使用,在無醫療人員協助情況下,無醫療知識的用戶也能無礙使用。因此,競賽評估標準為正確診斷,以及優越的用戶體驗。XPRIZE 基金會認為能夠達成競賽評估標準才能夠使 Tricorder 普及進而改善資源匱乏地區的醫療品質。
比賽宣布開始後 XPRIZE 基金會在 2013 年 9 月收到 312 隊來自 38 個國家報名,其中 29 隊在 2014 年 5 月送交了計畫書,在審核後 2014 年 10 月 XPRIZE 基金會宣布 10 隊入圍。最後在 2016 年 9 月各隊送交了開發的儀器,2016 年 10 月宣布六隊晉級準決賽,2016 年 12 月宣布兩隊進入決賽,第一名將於 2017 年 4 月 12 日在洛杉磯公布。
請參見官方提供 competition guidelines, competition timeline, and the two finalists.
比賽宣布開始後 XPRIZE 基金會在 2013 年 9 月收到 312 隊來自 38 個國家報名,其中 29 隊在 2014 年 5 月送交了計畫書,在審核後 2014 年 10 月 XPRIZE 基金會宣布 10 隊入圍。最後在 2016 年 9 月各隊送交了開發的儀器,2016 年 10 月宣布六隊晉級準決賽,2016 年 12 月宣布兩隊進入決賽,第一名將於 2017 年 4 月 12 日在洛杉磯公布。
請參見官方提供 competition guidelines, competition timeline, and the two finalists.
我們的團隊:動態生醫指標團隊
彭仲康教授在 2013 年為了參與比賽,成立了此跨領域、跨校也跨產業合作團隊。彭教授當時是從哈佛大學休假兩年,任中央大學生醫理工學院創院院長。HTC 的張智威博士應邀參與,借重其跨產學業界的經驗。
張博士加入 HTC 前在 Google 負責大數據研發,擔任 Director of Research 六年半。張博士曾任加州大學聖塔芭芭拉分校電機系正教授。再者,HTC 在軟硬體開發、工業設計、系統整合、用戶體驗領域有長足的經驗。有這些經驗才能夠成功的設計製造一個軟硬體結合雲平台大數據,且又能滿足比賽評估雙標準:重量限制和用戶體驗。
當然,此團隊必須仰賴醫學專家的指導。彭教授從臺大、北醫、清大、成大、中大、台大醫院邀請了表列六位醫學教授及醫師,擔任團隊不同疾病領域的指導。彭教授和這些醫學專家首先共同撰寫了參賽計畫書,憑此計畫書,動態生醫指標團隊在 2014 年 8 月進入了 XPRIZE 競賽前十名。從 2014 年 8 月起進入實際執行階段,張博士帶領 HTC 團隊先後投入六十位研究員、工程師、設計師,歷經兩年,完成了最後的產品 DeepQ。
當然,此團隊必須仰賴醫學專家的指導。彭教授從臺大、北醫、清大、成大、中大、台大醫院邀請了表列六位醫學教授及醫師,擔任團隊不同疾病領域的指導。彭教授和這些醫學專家首先共同撰寫了參賽計畫書,憑此計畫書,動態生醫指標團隊在 2014 年 8 月進入了 XPRIZE 競賽前十名。從 2014 年 8 月起進入實際執行階段,張博士帶領 HTC 團隊先後投入六十位研究員、工程師、設計師,歷經兩年,完成了最後的產品 DeepQ。
Name | Organization | Contribution |
---|---|---|
何奕倫 | 台大醫院 | 初賽顧問 |
林致廷 | 台灣大學 | 採血器與生物微感測器 |
劉明哲 | 北醫 | 臨床驗證 |
羅孟宗 | 中央大學 | 生理訊息 |
羅錦興 | 成功大學 | 尿液檢查 |
鄭桂忠 | 清華大學 | 生物晶片 |
DeepQ 產品介紹
DeepQ 包括五個主要診療系統:眼診、 血尿診、呼吸診、生命特徵診、問診。 這五個系統負責比賽疾病項目和生理訊號監控如下:
- 眼診:中耳炎、黑色素瘤。
- 血尿診:糖尿病、貧血、肺炎、白細胞增多症、尿道感染。
- 呼吸診:阻塞性肺疾病。
- 生命特徵診:
- 問診:藉著一個手機問診系統,診斷病人的可能疾病或沒有生病。
主要技術:HTC 大數據學習和轉移學習[1] 、王拔群醫師指導。
主要技術:傳統試紙技術,由 HTC 整合。(雖然專家學者們在計畫書中推薦使用微流道及生物晶片的技術、但是技術成熟度、重量限制和耗材貯存考慮、不適合居家使用。)
主要技術:HTC 開發。
生命特徵 :心率、呼吸頻率、體溫、氧飽和度、血壓。
疾病 :心律不整睡眠、呼吸暫停、高血壓。
主要技術:
心律不整睡眠、呼吸暫停:彭教授開發。
心率、呼吸頻率、體溫、氧飽和度、血壓、高血壓 :HTC。
主要技術:彭教授哈佛團隊 Dr. Andrew Ahn 提供技術指導,HTC 提供介面設計及 app 開發。HTC 在 2016 年繼續用人工智慧的技術增進準確度以及疾病診斷項目從 10 項到超過 100 項[2]。
團隊的貢獻
沒有彭教授和醫學專家的指導,動態生醫指標團隊不可能入圍前十名。HTC 在軟硬體開發、工業設計、系統整合、用戶體驗及大數據人工智慧的能力和經驗,將入圍計畫付諸實現。此跨領域、跨校也跨產業的團隊合作,為台灣醫療界和產業界的實力作了良好的見證。
附錄 1:論文
[1] Transfer representation learning for medical image analysis, CK Shie, CH Chuang, CN Chou, MH Wu, Edward Chang, IEEE Engineering in Medicine and Biology Society (EMBC), 2015.
[2] Inquire and Diagnose: Neural Symptom Checking Ensemble using Deep Reinforcement Learning, Kevin Tang, HaoCheng Kao, Jason Chou, Edward Chang, NIPS Deep Reinforcement Learning Workshop, 2016.
附錄 2:HTC 的貢獻
Area One. Artificial intelligence, big data analytics and transfer learning for disease diagnosis.
HTC developed AI algorithms for optical devices to detect otitis media and melanoma. HTC also developed algorithms to detect COPD and continuous measure blood pressure monitoring.
There are two major challenges to overcome when developing a classifier to perform automatic disease diagnosis. First, the amount of labeled medical data is typically very limited, and a classifier cannot be effectively trained to attain high disease-detection accuracy. Second, medical domain knowledge is required to identify representative features in data for detecting a target disease. Most computer scientists and statisticians do not have such domain knowledge. HTC uses deep learning and transfer learning to successfully address these two problems.
There are two major challenges to overcome when developing a classifier to perform automatic disease diagnosis. First, the amount of labeled medical data is typically very limited, and a classifier cannot be effectively trained to attain high disease-detection accuracy. Second, medical domain knowledge is required to identify representative features in data for detecting a target disease. Most computer scientists and statisticians do not have such domain knowledge. HTC uses deep learning and transfer learning to successfully address these two problems.
Area Two. Industrial design, system integration, cloud computing, wireless protocols, and user experience.
1. ID design for all instruments and a container hosting all with one single power supply, while keeping the total weight under five pounds.
2. Integrating various test strips into a tray and utilizing camera to read results in the cloud.
3. Developing intuitive easy-to-follow and error-proof designs for best user experience.
2. Integrating various test strips into a tray and utilizing camera to read results in the cloud.
3. Developing intuitive easy-to-follow and error-proof designs for best user experience.
Quantifying HTC’s Contributions w.r.t. Competition Scoring Criteria
I. To emphasize the importance of consumer use and adoption, only the highest scoring teams from the consumer experience evaluation will be eligible to win.
— 100% done by HTC
II. Scoring the highest across disease diagnostics, vital signs, and consumer experience.
The others such as blood/urine sense, optical sense, breath sense, were all done by HTC.
— 70% done by HTC
III. Pre-qualifying round proposal
— 10% done by HTC
— 100% done by HTC
II. Scoring the highest across disease diagnostics, vital signs, and consumer experience.
The others such as blood/urine sense, optical sense, breath sense, were all done by HTC.
— 70% done by HTC
III. Pre-qualifying round proposal
— 10% done by HTC